Predicting the Growth of AI
Scaling laws predicted the current explosion in AI capabilities. How useful are these laws going forward, and what do they tell us about the future now?
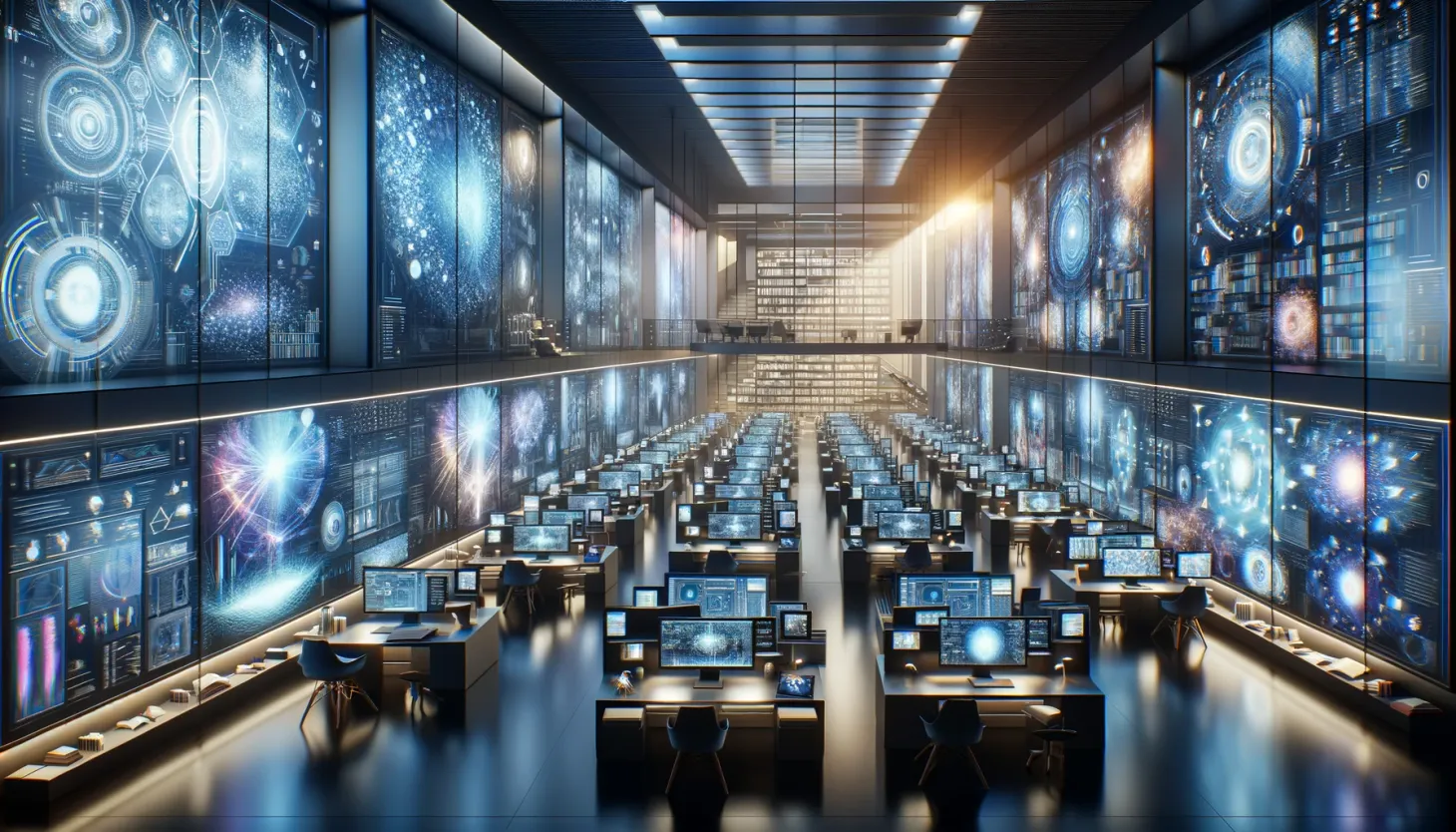
Beyond this wall lies a hidden archive.
Become a supporter to unlock it all.
SubscribeAlready a member? The door is open— come in.